How To Calculate A Rolling Average: A Clear Guide For Beginners
2024.09.16 20:16
How to Calculate a Rolling Average: A Clear Guide for Beginners
Calculating a rolling average is an essential tool for anyone who wants to analyze data over time. It is a statistical method that helps smooth out fluctuations in data by creating a series of averages of different selections of the full data set. Rolling averages are also referred to as moving averages, running averages, or rolling means.
A rolling average is calculated by taking the mean or average of a set of data points over a specific time period. The time period can be days, weeks, months, or years, depending on the data and the analysis goals. Rolling averages are commonly used in finance, economics, and marketing to track trends and identify patterns in data. They can also be used to forecast future trends based on historical data.
In this article, we will explore the different types of rolling averages, the benefits of using them, and how to calculate them using various methods. We will also provide examples of how rolling averages can be used in different industries and for different purposes. By the end of this article, you will have a clear understanding of what rolling averages are and how to use them to gain insights from your data.
Understanding Rolling Averages
Definition and Purpose
A rolling average, also known as a moving average or running average, is a statistical calculation used to analyze data points by creating a series of averages of different subsets of the entire dataset. The purpose of a rolling average is to smooth out fluctuations in the data and identify underlying trends over time.
Rolling averages are commonly used in finance, economics, and business to identify trends in stock prices, sales figures, and other key performance indicators. They are also used in scientific research to analyze trends in data collected over time.
Types of Rolling Averages
There are several types of rolling averages, each with its own unique characteristics and applications. The most common types of rolling averages include:
Simple Moving Average: This is the most basic type of rolling average, calculated by taking the average of a fixed number of data points over a specified time period.
Weighted Moving Average: This type of rolling average gives more weight to recent data points, resulting in a more responsive and accurate trend analysis.
Exponential Moving Average: This type of rolling average also gives more weight to recent data points, but uses a mathematical formula to give exponentially decreasing weight to older data points.
Cumulative Moving Average: This type of rolling average calculates the average of all data points up to a given point in time, providing a cumulative view of the data.
Each type of rolling average has its own advantages and disadvantages, and the choice of which type to use depends on the specific application and the characteristics of the data being analyzed.
Rolling averages are a powerful tool for analyzing trends in data over time. By understanding the different types of rolling averages and their applications, one can gain valuable insights into the underlying patterns and trends in the data.
Calculating a Simple Rolling Average
Calculating a rolling average is a common statistical analysis technique used to smooth out short-term fluctuations and highlight longer-term trends in data. A rolling average is also known as a moving average or running average. It is calculated by taking the mean of a set of values over a specified period, and then moving the window forward by one data point and recalculating the mean, repeating this process until all data points have been included in the calculation.
Selecting the Data Set
The first step in calculating a rolling average is to select the data set to be analyzed. The data set should be a time-series data set, where each data point represents a measurement taken at a specific point in time. The data set can be stored in a spreadsheet or a database, and should be sorted in chronological order.
Determining the Window Size
The window size is the number of data points that are included in the rolling average calculation. The window size should be chosen based on the frequency of the data and the desired level of smoothing. A larger window size will result in a smoother rolling average, but may also mask short-term fluctuations in the data. A smaller window size will result in a more responsive rolling average, but may be more susceptible to short-term fluctuations.
Calculating the Average
Once the data set and window size have been selected, the rolling average can be calculated using a simple formula. The formula for a simple rolling average is:
Rolling Average = (Sum of Values in Window) / (Window Size)
To calculate a simple rolling average in Excel, the AVERAGE function can be used with relative references. For example, to calculate a rolling average for a 3-day window, the formula in cell B2 would be:
=AVERAGE(A2:A4)
The formula can then be copied down the column to calculate the rolling average for each data point.
In conclusion, calculating a rolling average is a useful technique for smoothing out short-term fluctuations and highlighting longer-term trends in time-series data. By selecting the appropriate data set and window size, and using a simple formula, anyone can easily calculate a rolling average in Excel or any other spreadsheet software.
Weighted Rolling Average
A weighted rolling average is a type of rolling average that assigns different weights to each data point in the time series. The weights are determined based on the importance of each data point in the series. The weighted rolling average is useful when some data points are more significant than others.
Understanding Weighting
In a weighted rolling average, the weights assigned to each data point determine the relative importance of each point in the calculation of the average. The weights can be assigned based on criteria such as time, frequency, or importance. For example, if a company wants to calculate the average daily sales for the last 30 days, it may assign a higher weight to the more recent data points, as they are more relevant to the current state of the business.
Assigning Weights to Data Points
To assign weights to data points, you need to determine the criteria for assigning the weights. For example, if you want to calculate the weighted rolling average of a stock price, you may assign a higher weight to the closing price of the current day and a lower weight to the closing price of the previous day. The weights can be assigned using a formula or a table.
Weighted Average Calculation
To calculate the weighted rolling average, you need to multiply each data point by its weight and then divide the sum of the products by the sum of the weights. The formula for calculating the weighted rolling average is:
Weighted Average = (w1 * x1 + w2 * x2 + ... + wn * xn) / (w1 + w2 + ... + wn)
where w1, w2, ..., wn are the weights assigned to data points x1, x2, ..., xn.
In Excel, you can calculate the weighted rolling average using the SUMPRODUCT function. The function multiplies each data point by its weight and then sums the products. The result is divided by the sum of the weights. For example, the formula for calculating the weighted rolling average of a range of data in Excel is:
=SUMPRODUCT(weights, data) / SUM(weights)
where weights is the range of weights assigned to each data point, and data is the range of data points.
Overall, the weighted rolling average is a useful tool for analyzing time series data when some data points are more significant than others. By assigning weights to each data point, you can calculate a more accurate average that reflects the current state of the business.
Using Rolling Averages in Data Analysis
Rolling averages are a powerful tool in data analysis that can help identify trends and patterns in a dataset. By calculating the rolling average of a dataset, you can smooth out the fluctuations in the data and highlight underlying trends that may not be immediately apparent.
Trend Identification
One of the most common uses of rolling averages is to identify trends in a dataset. By calculating the rolling average over a specific time period, you can identify whether the data is trending upwards or downwards. This can be particularly useful in financial analysis, where investors use rolling averages to identify trends in stock prices.
For example, if you are analyzing sales data for a company, you could calculate the rolling average of sales over the past 12 months. By plotting this data on a graph, you can easily identify whether sales are increasing or decreasing over time. This can help you make informed decisions about future business strategies.
Data Smoothing
Another benefit of using rolling averages is that they can help smooth out the fluctuations in a dataset. This can be particularly useful when analyzing data that is subject to seasonal or cyclical variations. By calculating the rolling average over a longer time period, you can smooth out the impact of these variations and highlight underlying trends in the data.
For example, if you are analyzing website traffic data, you may notice that traffic spikes on weekends and dips during the week. By calculating the rolling average over a longer time period, you can smooth out these fluctuations and identify whether overall traffic is increasing or decreasing.
Overall, rolling averages are a powerful tool in data analysis that can help identify trends and patterns in a dataset. By using rolling averages to smooth out fluctuations in the data, you can highlight underlying trends and make informed decisions about future business strategies.
Rolling Average Limitations
Lag Effect
A rolling average is a useful tool to analyze data over time. However, it has a limitation known as the lag effect. This means that the rolling average will always lag behind the actual data. The degree of lag depends on the window size used in the calculation.
For example, suppose you are calculating a rolling average of monthly sales data for a business. If you use a window size of three months, the rolling average for January will be based on the sales data from November, December, and January. This means that the rolling average for January will not reflect any changes in sales that occurred in February or March.
Choosing an Appropriate Window Size
Choosing an appropriate window size is crucial when calculating a rolling average. A small window size will result in a rolling average that is highly sensitive to fluctuations in the data, while a large window size will result in a rolling average that is less sensitive to fluctuations but may not reflect recent changes in the data.
When choosing a window size, it is important to consider the purpose of the analysis and the characteristics of the data. For example, if the data is highly volatile, a smaller window size may be more appropriate to capture short-term changes. On the other hand, if the data is relatively stable, a larger window size may be more appropriate to capture longer-term trends.
In summary, while a rolling average is a useful tool for analyzing data over time, it is important to be aware of its limitations, such as the lag effect and the need to choose an appropriate window size. By understanding these limitations and selecting an appropriate window size, analysts can make better use of rolling averages to gain insights into their data.
Implementing Rolling Averages with Software
Rolling averages can be easily implemented with software. Here are some ways to do it using spreadsheets and programming languages.
Spreadsheets
Spreadsheets such as Microsoft Excel and Google Sheets have built-in functions for calculating rolling averages. The AVERAGE function can be used to calculate the average of a range of cells. To calculate a rolling average, a range of cells can be selected, and the AVERAGE function can be used with the OFFSET function to shift the range by a certain number of cells.
For example, to calculate a rolling average of the last 3 cells in column A, the following formula can be used:
=AVERAGE(OFFSET(A1,COUNTA(A:A)-3,0,3,1))
This formula uses the COUNTA function to count the number of non-empty cells in column A, and Calculator City the OFFSET function to shift the range by that number minus 3 cells. The AVERAGE function is then used to calculate the average of the last 3 cells in the shifted range.
Programming Languages
Programming languages such as R and Python have libraries for calculating rolling averages. In R, the zoo package provides the rollmean function for calculating rolling means. The rollmean function takes a vector of values and a window size as input, and returns a vector of rolling means.
Here is an example of using the rollmean function to calculate a rolling average of a vector of values:
library(zoo)
values -lt;- c(1, 2, 3, 4, 5)
rolling_mean -lt;- rollmean(values, k = 3, align = "right")
In this example, the vector of values is [1, 2, 3, 4, 5], and the window size is 3. The align parameter is set to "right" to align the rolling means with the last value in the window.
In Python, the pandas library provides the rolling function for calculating rolling statistics. The rolling function takes a pandas series or dataframe as input, and returns a rolling object that can be used to calculate rolling statistics.
Here is an example of using the rolling function to calculate a rolling average of a pandas series:
import pandas as pd
values = pd.Series([1, 2, 3, 4, 5])
rolling_mean = values.rolling(window=3).mean()
In this example, the pandas series is [1, 2, 3, 4, 5], and the window size is 3. The mean function is used to calculate the rolling mean.
Advanced Applications
Rolling Averages in Time Series Forecasting
Rolling averages are commonly used in time series forecasting to smooth out fluctuations and identify trends. By calculating the rolling average of a time series, analysts can better understand the underlying patterns and make more accurate predictions.
One of the most popular methods for calculating rolling averages in time series forecasting is the moving average method. This involves calculating the average of a fixed number of data points over a rolling window. For example, a 3-month moving average would be calculated by taking the average of the last three months of data.
Another method for calculating rolling averages in time series forecasting is the exponential moving average (EMA) method. This method gives more weight to recent data points, which can be useful in situations where recent trends are more important than historical trends.
Rolling Averages in Financial Markets
Rolling averages are also commonly used in financial markets to identify trends and make investment decisions. In particular, the 50-day and 200-day moving averages are widely used by traders and analysts to identify long-term trends in stock prices.
When the price of a stock is above its 50-day moving average, it is considered to be in an uptrend, while when the price is below its 50-day moving average, it is considered to be in a downtrend. Similarly, when the price of a stock is above its 200-day moving average, it is considered to be in a long-term uptrend, while when the price is below its 200-day moving average, it is considered to be in a long-term downtrend.
Rolling averages can also be used in conjunction with other technical indicators, such as the Relative Strength Index (RSI) and Moving Average Convergence Divergence (MACD), to make more informed investment decisions. By using rolling averages in this way, traders and analysts can better understand the underlying trends in financial markets and make more accurate predictions about future price movements.
Frequently Asked Questions
What is the difference between a rolling average and a moving average?
A rolling average and a moving average are terms that are often used interchangeably, but they do have some differences. A rolling average is a type of moving average that is used to analyze data points by creating a series of averages of different subsets of the full data set. A moving average, on the other hand, is a type of statistical analysis that is used to analyze a set of data points by creating a series of averages of different subsets of the full data set, with each subset being the same size.
How can you compute a 12-month rolling average?
To compute a 12-month rolling average, you will need to take the sum of the data for the past 12 months and divide it by 12. This will give you the average for the past 12 months. As each new month's data becomes available, you can add it to the sum and drop the oldest month's data to keep the rolling average to 12 months.
What steps are involved in calculating a 7-day rolling average?
Calculating a 7-day rolling average involves taking the sum of the data for the past 7 days and dividing it by 7 to get the average for the past 7 days. As each new day's data becomes available, you can add it to the sum and drop the oldest day's data to keep the rolling average to 7 days.
How do you implement a rolling average in Power BI?
To implement a rolling average in Power BI, you can use the DAX formula AVERAGEX to calculate the average of a column over a specified time period. You can use the FILTER function to specify the time period, and the CALCULATE function to calculate the average.
What is the formula to determine a 3-month rolling average?
To determine a 3-month rolling average, you will need to take the sum of the data for the past 3 months and divide it by 3. This will give you the average for the past 3 months. As each new month's data becomes available, you can add it to the sum and drop the oldest month's data to keep the rolling average to 3 months.
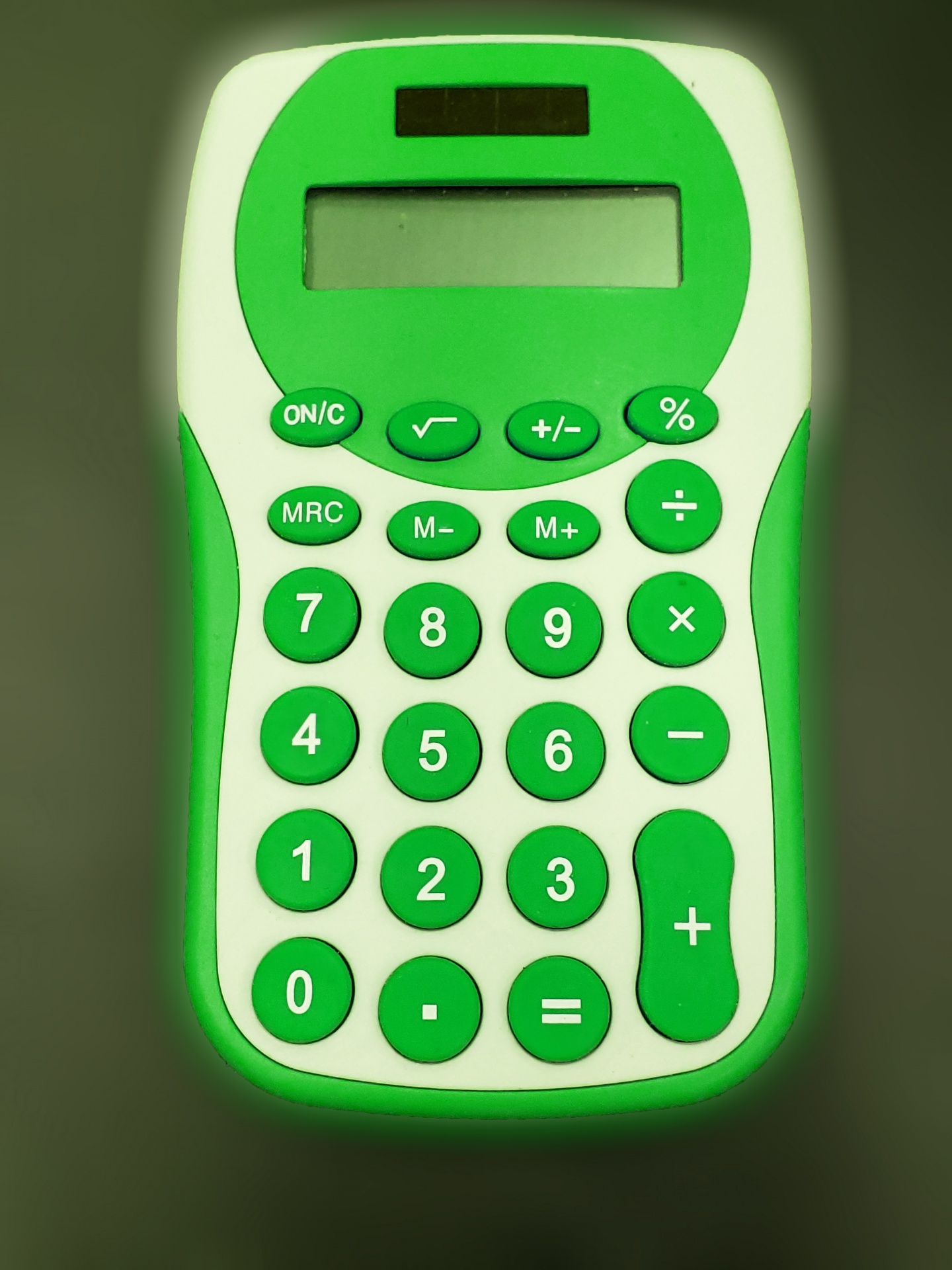
Why might one opt to use a rolling average in data analysis?
A rolling average can be useful in data analysis because it allows you to smooth out fluctuations in data over time, making it easier to identify trends and patterns. It can also help to remove the effects of seasonality and other short-term fluctuations in the data, making it easier to see the underlying long-term trends.